Data remains the key enabler for achieving these goals. However, despite significant investments in data acquisition and storage, the ability to convert raw data to meaningful and actionable insights is far from reaching its full potential. While new analytics technologies provide a mechanism for transforming data into insights, these results can only be unlocked with a sufficiently trained workforce to understand the inputs and interpret results.
The shortage of skilled labour in the oil and gas industry is well-known, and it presents a fundamental challenge. This skills gap is partly due to an ageing workforce – with many of the most highly skilled employees nearing retirement – and the industry simultaneously losing new and existing talent to more popular fields, such as renewables. Investments in new technologies like advanced analytics, AI, and machine learning require organisations to continually upskill the remaining workforce, which can be difficult due to time and other resource constraints.
This article dissects both the need for and challenges of upskilling workers in the oil and gas sector, and it explains how technology partners are supporting and accelerating workforce empowerment and enablement to help companies overcome these difficulties.
Skilled shortage in industry
The skilled labour shortage is not unique to the oil and gas industry. The World Economic Forum’s Future of Jobs Report 2023 found that six in ten workers will require training before 2027, but only half of workers have access to adequate training opportunities today. The same report found in all industries collectively, training workers to use AI and big data ranks third among company upskilling priorities over the next five years.
However, oil and gas is lagging other sectors, particularly in adopting cutting-edge technologies. The 2024 Global Energy Talent Index (GETI) Report found that the oil and gas industry has the lowest rates of AI adoption. This is partially attributed to a lack of employee support, with 32% of respondents stating that they worry about inadequate training leading to misuse or poor adoption.
This finding is significant because several cross-industry surveys are finding that large salaries are no longer enough to retain employees. Workers recognise that longevity in a sector and their future employability depend on access to and successful adoption of new technologies. According to the GETI report, 87% of workers in the oil and gas sector would consider switching jobs, with 50% citing professional growth and learning opportunities in a role as a key priority.
As a result, companies who fail to either invest in new technologies or empower their workforce to successfully adopt the tools are at risk of both losing their competitive edge and exacerbating an already problematic skill shortage.
Advanced analytics for workforce empowerment
The good news is the reverse is also true: companies who diligently invest in modern technologies and strong upskilling programmes can expect measurable improvements in terms of productivity and efficiency, while also attracting and maintaining a motivated and energised workforce.
Many advanced analytics platforms provide the ability to access multiple disparate data sources so users can seamlessly combine and interrogate data regardless of its origin. This capability – in combination with intuitive self-service tools, which are designed for time-series data analysis – empowers subject matter experts (SMEs) to translate their raw data into valuable insights.
Access to these platforms is transformative because it allows users to make tangible impacts in their organisation, such as increasing uptime, completing root cause analyses, or monitoring greenhouse gas emissions in real time. Despite the relative ease of using these tools, there is a notable requirement for training – both in leveraging the technology’s functions and features, and in the principles of data analytics.
Traditionally, training was often a time-consuming activity that required multiple days off-site, and its effectiveness was contingent upon numerous factors that were tough to control. To address these limitations, some technology companies are pioneering new ways of upskilling the workforce, improving adoption with more time focused on value-adding activities.
Generative AI to boost productivityOne clear example of this is the recent upsurge in companies investing in generative AI (GenAI). This technology offers, among many other possibilities, a new opportunity for workers to obtain faster results, while reducing the need for formal education and training. Many embedded and stand-alone solutions are available that provide a way to generate text or code based on a user’s prompt (Figure 1). For engineers who have not had formal opportunities to study programming languages like Python or R, this feature significantly lowers the barrier to entry, and it facilitates better understanding and collaboration with data scientist colleagues or third parties.
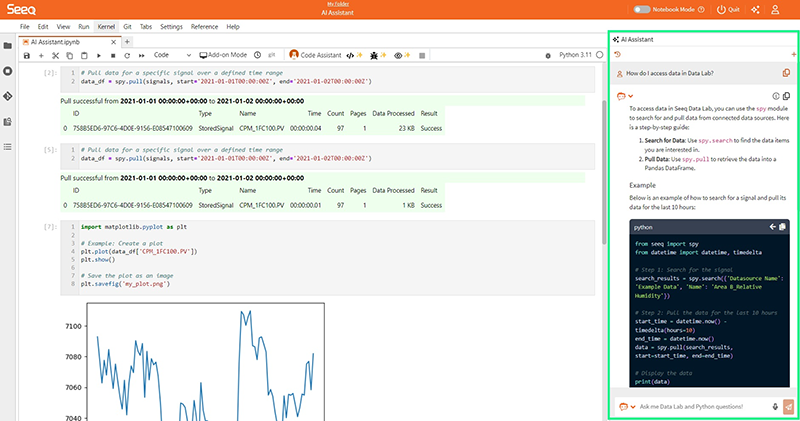
One national energy company found that by providing engineers with a GenAI assistant within their existing advanced analytics platform, Seeq, they were able to accomplish a task in-house in just 15 minutes, that previously required more than four days and support from an outside coding team. Similarly, companies Ascend Performance Materials and British Sugar reported that AI assistance in the same platform has enabled their SMEs to cut analysis time in half, empowering teams to deliver more business value from their data. When generative technologies are used to accelerate the implementation of well-known and understood techniques or methodologies, productivity gains are clear. However, when these tools are used to develop new or more complex use cases, it must be noted that providing access to these generative capabilities does not necessarily yield widespread adoption or satisfactory results.
Throughout industrial and commercial landscapes, there is, rightfully, an inherent scepticism in GenAI results, and the presence of hallucinations is a clear barrier to adoption. Without adequate training and visibility of successful use cases, workers are typically hesitant to invest time in learning a new technology, or they may not trust the results and abandon their use. Particularly in the oil and gas industry – where analyses inform decisions with huge safety, operational, and environmental consequences – it is essential that training keeps pace with technological development.
It is not reasonable to treat AI or generative technologies as a magic bullet that solve all operational issues. This is especially true in the process industries, which have an intrinsic need for data cleansing and contextualisation due to the presence of sensor noise, measurement errors and drift, and distinct operating modes. Users must be equipped with the knowledge and the ability to prepare their data effectively, as shown in Figure 2.
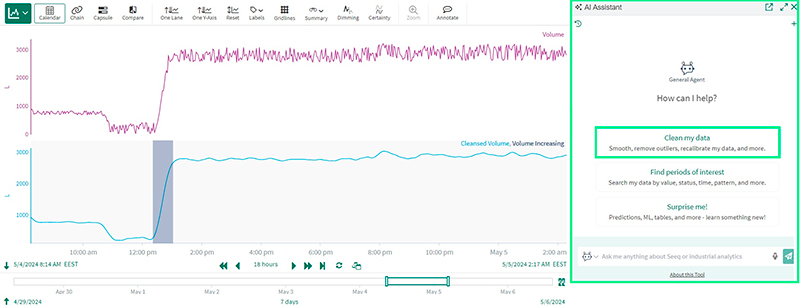
Technology’s output is only as good as the quality of the data, and as the saying goes, garbage in equals garbage out.
When it comes to interpreting the results, end-users must also be trained on fundamental principles, such as how to validate results. When more workers are enabled to deploy complex machine learning algorithms using simple prompts, it is critical to precede that with the knowledge, understanding, and ability to evaluate the models’ validity. Pairing every new technological deployment with a comprehensive and well-defined training path is of the utmost importance.
Workers need sufficient training in these fundamental areas to achieve success with advanced machine learning techniques and AI implementations. The good news is that with the emergence of remote and self-paced e-learning training, materials are more accessible than ever in employees’ daily workflows (Figure 3). Not only do e-learning paths make training opportunities more accessible, but research has also shown that learning through experience yields better results than traditional classroom instruction, emphasising the importance of access to resources while the work is being performed. When implemented effectively, this capability substantially enhances the likelihood of successful adoption.
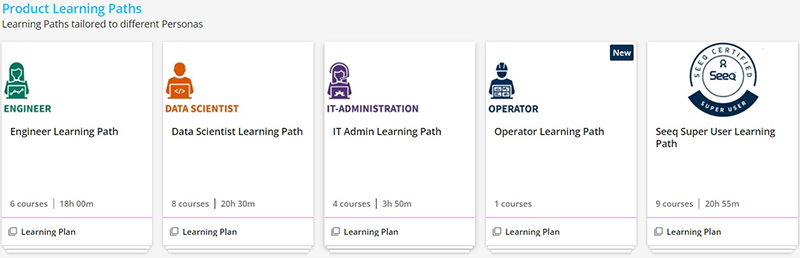
By following a structured adoption plan from its data analytics platform provider, one US multinational oil and gas company rolled out AI-informed advanced analytics technology to more than 50 sites globally. As adoption increased and experts in the technology emerged, the company followed a programme known as ‘train the trainer’. This method prioritises empowering internal champions, who understand where the technology should be implemented, to facilitate training and share successes with new learners.
This community-led approach has resulted in over 4000 unique users per month who are now equipped with the knowledge to identify process anomalies, monitor equipment, predict failures, and more. The company’s success with this method showcases the benefit of structured training roll-out planning. Additionally, establishing communities of practice, or mentor programmes, can rapidly accelerate enterprise-level technology adoption.
Workflow solutions for enterprise-level monitoring
Armed with generative functionalities, new advanced analytics techniques, and scalable platforms, organisations that embrace new technologies can expect an increase in both the complexity and scale of their data-driven initiatives. While this facilitates rapid operational improvement, it also inevitably results in the need for new workflows. Flint Hill Resources’ (FHR) monitoring department quickly realised this, as it was tasked with remote surveillance of two refineries to identify early risks and proactively review asset health across thousands of unique assets.
The company required a structured workflow to effectively monitor the many thousands of alerts generated so users could effectively triage, manage, and monitor at scale. Although the method for detecting anomalies was designed and implemented by a centralised team, facility operators and engineers with additional insights and knowledge about the process were the most frequent application end users. In partnership with Seeq, FHR deployed an industrial enterprise monitoring application that enabled users to quickly evaluate whether action was required based on any anomaly detected. The solution crucially provided the ability to capture feedback as well, ensuring models remain accurate and relevant (Figure 4).
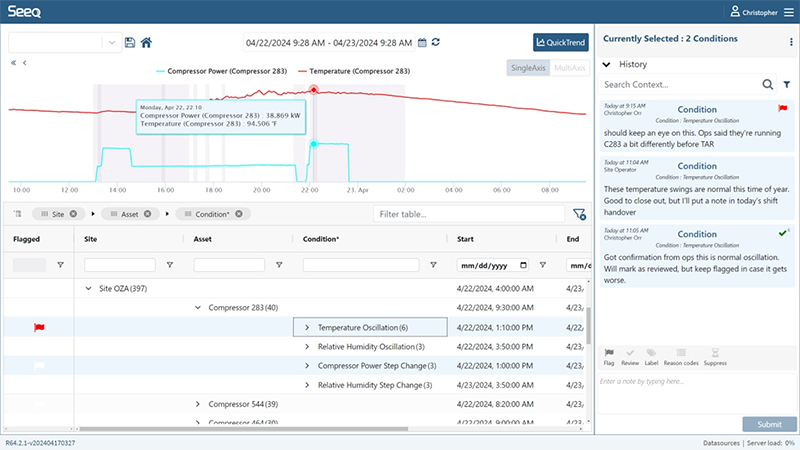
For example, new operating conditions can increase the incidence of false positive alerts over time. An onsite engineer with additional context can flag these alerts accordingly, and either suppress them or collaborate with the monitoring team to reduce further occurrences. This collaborative workflow was essential in FHR’s monitoring objectives, and it has enabled the company to efficiently monitor over 8000 parameters and 4000 assets.
In the context of AI and advanced analytics techniques, users require structured workflows to continually evaluate and provide feedback regarding the accuracy and reliability of the data insights they are provided, regardless of technique complexity – be it a simple univariate threshold alert, or a complex machine learning algorithm. With a lack of trust most frequently cited as the primary challenge for adopting new technologies in the oil and gas industry, this feedback loop is a crucial component for successful deployment, and it ultimately results in better business outcomes.
Investing in upskilling for organisation-wide benefits
The oil and gas industry is facing a myriad of challenges to stay profitable while adhering to increasingly strict environmental regulations. While new technologies, such as advanced data analytics platforms and generative technologies, offer opportunities to improve productivity, they also introduce new training and adoption requirements.
Regardless of the technology relied on for process and data insights, the fundamental enabler is a skilled workforce capable of understanding, validating, and acting on the results. This means companies must heavily invest in upskilling their workforce, and provide clear guidelines for the use of generative and other advanced data technologies.
Organisations that fully commit to both workforce empowerment and modern technological tools will experience the highest return on their investments, and opportunities to work with cutting-edge technologies support talent acquisition and retainment. This approach enables organisational shifts where innovation flourishes to improve productivity, achieve sustainability goals, and maintain a competitive edge.
Written by Fiona Guinee, Senior Analytics Engineer at Seeq.